AI-based systems, especially those based on machine learning technologies, have become central in modern societies. In the meanwhile, users and legislators are becoming aware of privacy issues. Users are increasingly reluctant to share their sensitive information, and new laws have been enacted to regulate how private data is handled (e.g., the GDPR).
Federated Learning (FL) has been proposed to develop better AI systems without compromising users’ privacy and the legitimate interests of private companies. Although still in its infancy, FL has already shown significant theoretical and practical results making FL one of the hottest topics in the machine learning community.
Given the considerable potential in overcoming the challenges of protecting users’ privacy while making the most of available data, we propose a workshop on Federated Learning Technologies (FLT) at TheWebConf 2023.
This workshop aims to focus the attention of the TheWebConf research community on addressing the open questions and challenges in this thriving research area. Given the broad range of competencies in the TheWebConf community, the workshop will welcome foundational contributions and contributions expanding the scope of these techniques, such as improvements in the interpretability and fairness of the learned models.
Call for Papers
The workshop will be centered on the theme of improving and studying the Federated Learning setting. It will welcome applicative and theoretical contributions as well as contributions about specific settings and benchmarking tools. The topics include (but are not limited to):
- Federated Learning with non-iid data distributions;
- Security and privacy of FL systems (e.g., differential privacy, adversarial attacks, poisoning attacks, inference attacks, data anonymization, model distillation, secure multi-party computation, …);
- Other non-functional properties of FL (e.g., fairness, interpretability/explainability, personalization, …);
- Algorithmic and theoretical advances in FL;
- FL variants and Decentralized Federated Learning (e.g., vertical FL, split learning, gossip learning, …);
- Applications of FL (e.g., FL for healthcare, FL on edge devices, advertising, social network, blockchain, web search, …);
- Tools and resources (e.g., benchmark datasets, software libraries, …).
Workshop papers should not exceed 12 pages in length (maximum 8 pages for the main paper content + maximum 2 pages for appendixes + maximum 2 pages for references). Papers must be submitted in PDF format according to the ACM template published in the ACM guidelines, selecting the generic “sigconf” sample. The PDF files must have all non-standard fonts embedded. Workshop papers must be self-contained and in English.
Schedule (tentative)
Time | Event | Speaker |
---|---|---|
9:00 – 9:05 | Opening | Workshop Organizers |
9:05 – 9:50 | Keynote | Prof. Marco Aldinucci, University of Turin, Italy High-Performance Computing and Federated Learning |
9:50 – 10:30 | Hands-on session | Walter Riviera, Intel Hands-on session with Open FL |
10:30 – 10:45 | Coffee break | |
10:45 – 11:45 | Paper presentations | Yifei Zhang, Dun Zeng, Jinglong Luo, Zenglin Xu and Irwin King. A Survey of Trustworthy Federated Learning with Perspectives on Security, Robustness, and Privacy Gianluca Mittone, Filip Svoboda, Marco Aldinucci, Nicholas D. Lane and Pietro Liò. A Federated Learning Benchmark for Drug-Target Interaction Yao Chen, Shan Huang, Wensheng Gan, Gengsen Huang and Yongdong Wu. Federated Learning for Metaverse: A Survey Sumam Francis, Kanimozhi Uma and Marie-Francine Moens. Understanding the Impact of Label Skewness and Optimization on Federated Learning for Text Classification |
11:45 – 12:25 | Hands-on session | Dr. Chaoyang He, CEO of FedML Hands-on session with FedML |
12:25 – 12:30 | Concluding remarks | Workshop Organizers |
Workshop organizers
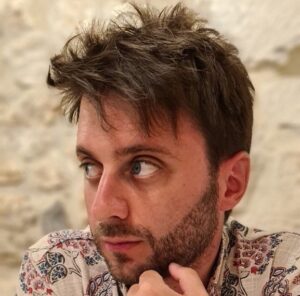
Mirko Polato
Assistant Professor
Department of Computer Science, University of Turin, Italy
mirko.polato[at]unito.it
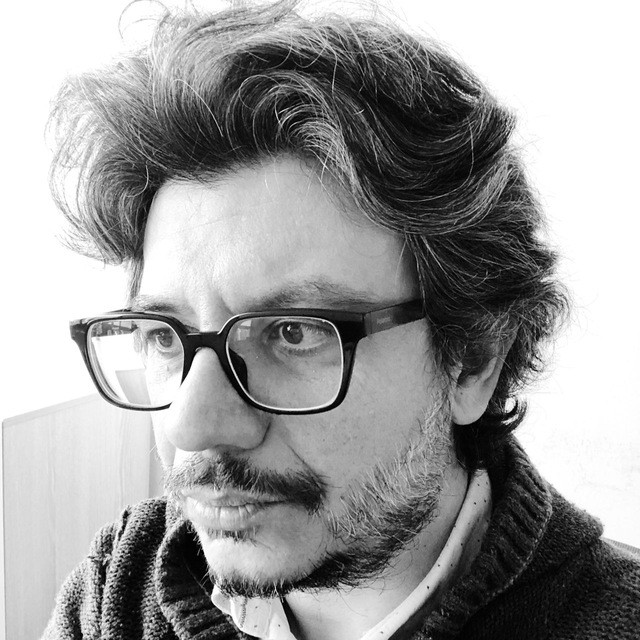
Roberto Esposito
Associate Professor
Department of Computer Science, University of Turin, Italy
roberto.esposito[at]unito.it
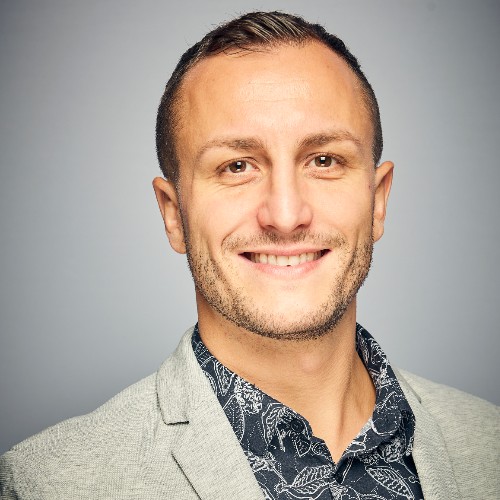
Walter Riviera
AI Technical Lead EMEA (Data centre Group)
Intel Corporation, Swindon, UK
walter.riviera[at]intel.com
PhD Student
University of Verona, Italy
walter.riviera[at]univr.it
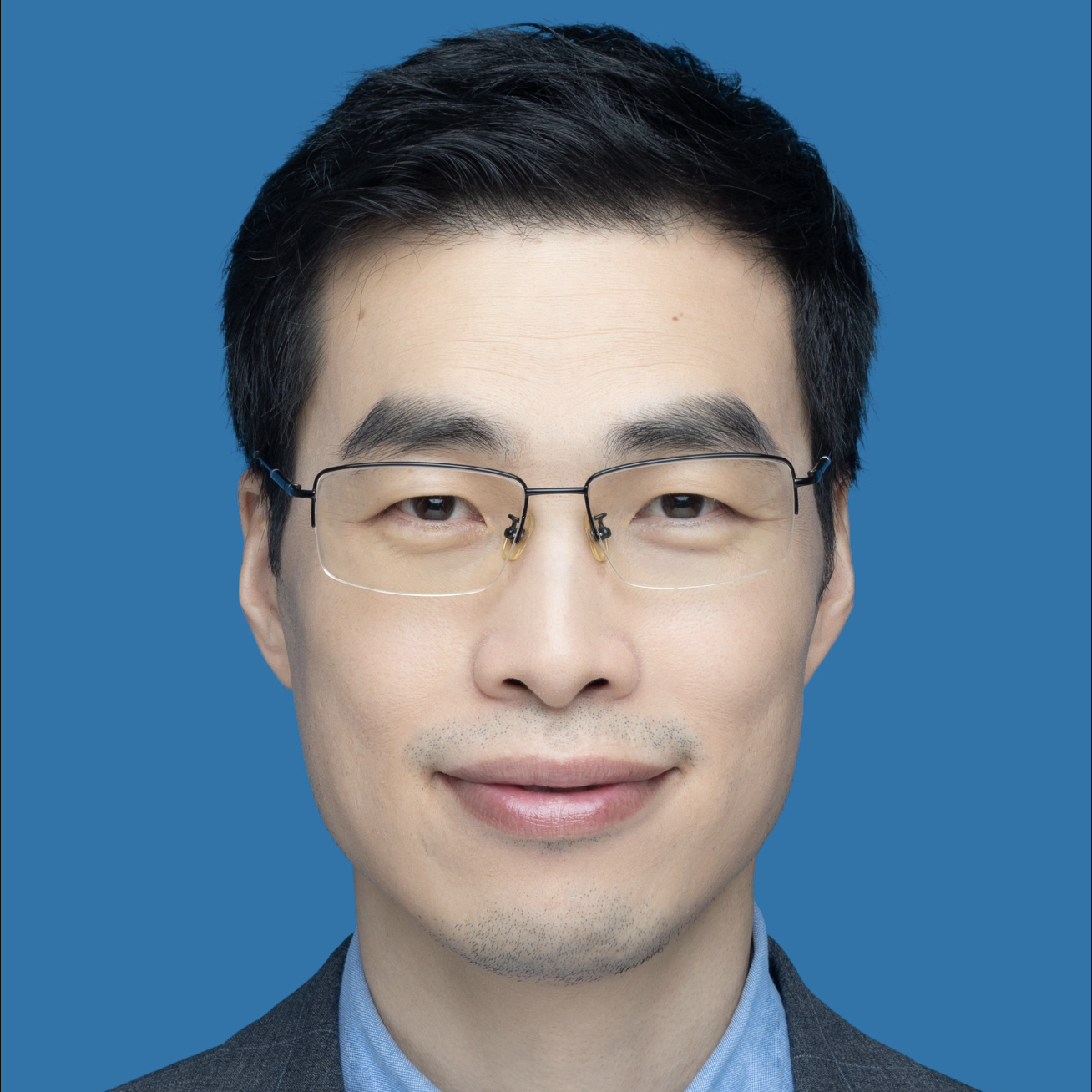
Xu Zenglin
Full Professor
Harbin Institute of Technology, Shenzhen
zenglin[at]gmail.com
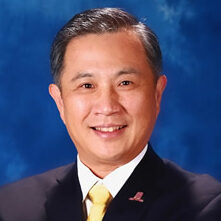
Irwin King
Full Professor
The Chinese University of Hong Kong, Hong Kong
irwinking[at]gmail.com
Venue
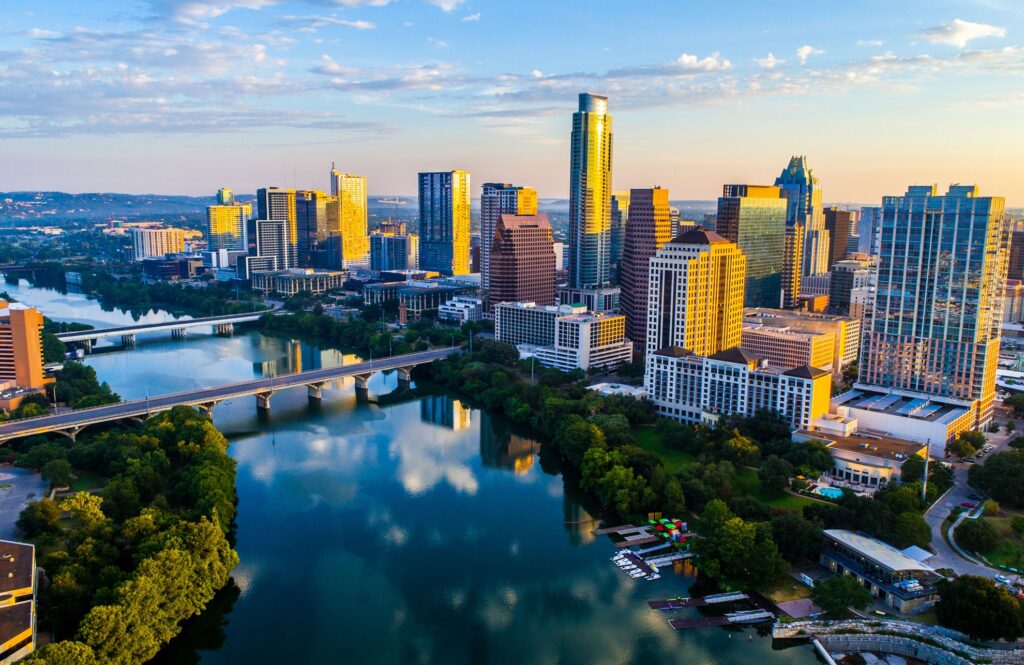
Funding
This event was partially funded by:
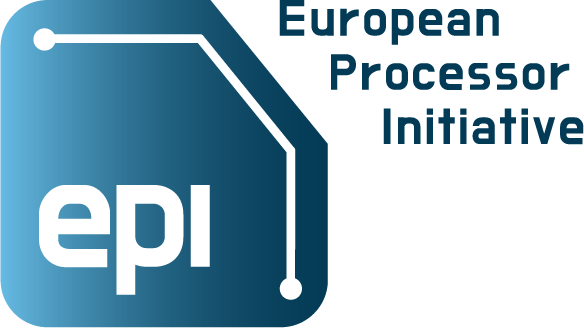
The EPI project has received funding from the European High Performance Computing Joint Undertaking (JU) under Framework Partnership Agreement No 800928 and Specific Grant Agreement No 101036168 (EPI SGA2). The JU receives support from the European Union’s Horizon 2020 research and innovation programme and from Croatia, France, Germany, Greece, Italy, Netherlands, Portugal, Spain, Sweden, and Switzerland.

The European PILOT project has received funding from the European High-Performance Computing Joint Undertaking (JU) under grant agreement No.101034126. The JU receives support from the European Union’s Horizon 2020 research and innovation programme and Spain, Italy, Switzerland, Germany, France, Greece, Sweden, Croatia and Turkey.